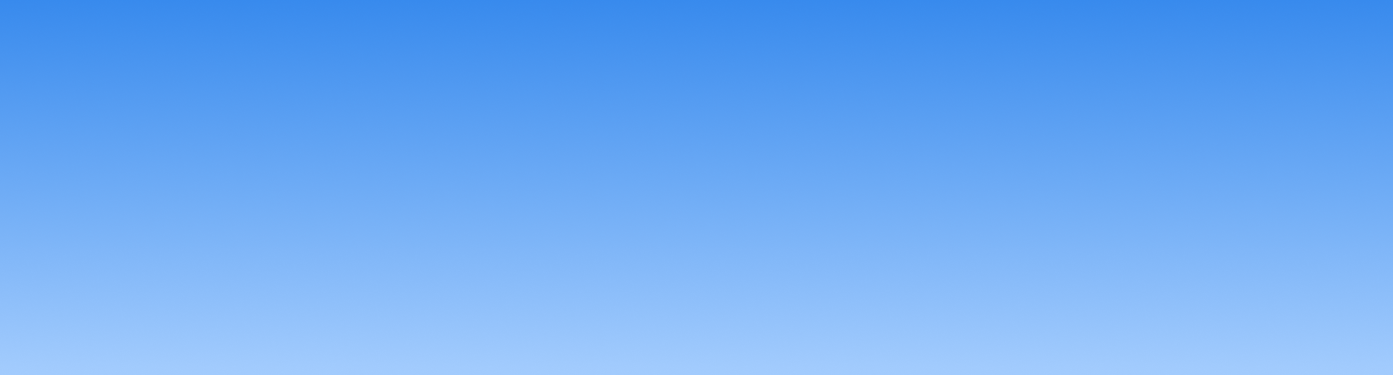
Data visualization for AI-generated marketing report
Duration: 1 month| Role: UX Designer |Team: Katie Hoang (UX design), Zach Holloway (PM), Melinda Weathers (Principal Software engineer)
Tools used: Figma | Methods: Stakeholder feedback facilitation, Lo-fidelity wireframes, user research, market research, and prototyping
Problem
Small medium business users (SMB) need to know what campaigns can be accurately attributed to the many touch points a customer has before scheduling an appointment or visiting a business’ site. This includes offline, online, and referral sources that all influence leads to visit a site and convert.
With AI, CallRail’s LLM is able to extract SRA, self reported attribution. SRA captures where customers found the business as they say it explicitly or vaguely in a call. The value-add is that first-touch attribution is accurate and the relationship of this within a customer journey to depict the many touch points a customer interacts with is represented for higher quality reporting.
The report received low engagement, low discoverability, and didn’t improve a concerning churn rate of the product. This caused concern for more investigation to iterate on this release.
Discover
I audited the experience of the report via UX heuristics and primary and secondary user research to tailor the solution to a marketer’s needs to best leverage how SRA fits into their workflow.
I synthesized user research findings from live feedback sessions where I asked customers how they use the SRA report today.
Here are some of the questions and answers from real users:
Q: Does SRA bring value to you?
A: “It is what it is”
A: “Digging around for 6-8 weeks so far. I have not pulled it into actionable insights so far”
Q: If you could make SRA more useful, what would you do?”
A: “Can you help me identify which source helped drive this traffic so I know who to thank or where to invest more in?”
A: "I don’t know why it is so far removed from the data recorded on each call already”
Takeaways <> Requirements
The 5 interviews we had informed the requirements below:
Users need to know trends and performance of SRA to proactively improve their marketing strategy.
First-touch attribution is different from a first-touch attribution model and needs to be differentiated in the app
Users already go to certain places in the app to classify their calls and leads today. SRA needs to belong in those places to improve the attribution picture.
*Users lean on organic marketing to boost their local listing in google business profile. Organic marketing is the best way to grow a business reach because it is free
*This was an insight I gleaned and advocated to be part of the requirements to my PM based on secondary research
Challenges
Can’t make financial recommendations
CallRail does not house any financial data about how much users spend on their campaigns.
How might the data visualization provide impact in a way that doesn’t suggest recommendations on budgeting, but rather sourcing more of the data to improve the LLM?
Adding SRA to a crowded UI in recorded calls
Customer spoken data already exists in CallRail. The app surfaces call highlights and key terms spoken in every call.
How might adding SRA data fit in the current UI in a way that won’t be overlooked?
Ideation
This project was two fold. It required adding data visualization on the SRA report and creatively add SRA in the context of the full customer attribution journey and individual call where it is sourced
To meet the needs of users:
For the report:
Callout trends, validate performance, and optimization opportunities in the data that can be relevant to improving their marketing strategy
For where users view attribution data today in other parts of the app:
Clearly label SRA when it is found in a recorded call so it can be tracked by the interaction and in context to the customer journey. This gives marketers a competitive advantage by knowing how a robust campaign strategy is valuable to implement versus a
UX inspo
To leverage existing user knowledge of software, I like to search up standard practices of when to use what elements in design to help flatten the learning curve of adopting software
UX/UI inspo from Youtube
Youtube is not designing for the same user as CallRail, but they are prioritizing a similar JTBD, which is showing users aggregate data to suggest trends, validate users where they are successful, and suggest areas of improvement.
Lo-fi’s for stakeholder feedback
These were the lo-fi’s I felt most strongly about in achieving the user goal. I shared these with stakeholders and design peers in a quick turn around to get their feedback and alignment on both.
Report lo-fi
Pro - Calling out Organic SRA, Calls with SRA, and Qualified leads with SRA. These are important individually to call out because of their significant impact on business growth and marketing ROI.
Feedback - Would ignoring paid SRA be dismissive of the value agencies bring to SMBs too?
The bar chart shows performance in a given time, but what about trends?
Call interaction lo-fi (low visibility)
Pro - Recycling the current UI layout of the call interaction card to reduce development costs.
Feeback - Users will not be able to notice this callout to SRA with no visual hierarchy of importance or use of grouping and it is not an impactful solution
Report lo-fi
Pro - Visually interesting way to show SRA data. Breaking apart from the many pie charts the app uses to display data today helps users know this is new and they spend more time to discover its value
SRA and SBA relationship are both 2 sides of the same attribution coin. Showing them together achieves marketing accuracy.
Feedback - A word bubble is not a user friendly way to quickly show trends in the case that a lot of the SRA is around the median count
Call interaction lo-fi (high visibility)
Pro - Improving scan-ability of call insights to speed up call interaction analysis
Grouping all AI features together to show value add and feeling of up to date tools that enhance workflow
Feedback - This will increase development effort due to reshifting of component. This component is used in 3 different places, so extensive QA is required during development.
Alignment
I had to share the lo-fi designs with the AI-team, which includes PM and a lead designer, as well as the larger product team with stakeholders, like the VP of design and VP of product.
Some feedback/questions I received related to the implementation included:
Feedback: “We have agency customers who show their value to clients through paid marketing sources. Omitting that here is a risk to losing them as customers”
Action taken - I swapped out qualified lead with paid sources because it is vital that we remain a tool for agencies to prove their marketing ROI to their SMB clients.
Feedback: “Is adding SRA data to the person timeline (high visibility) an over approximation on the value SRA has for users? Are we sure users want to know SRA data as part of a lead journey or as part of an aggregate instead?
Action taken - Segmenting this data already leads to confusion, as noted in customer interviews, and leads to inaccurate reporting on how a robust marketing mix holistically brings in leads. It is important we consider that a companies marketing strategy is dependent on how accurately we display the information of it, so customers can adjust their budget as they see fit.
Design and prototype
After iterating on feedback and explaining my rationale to uphold my design decisions, these are the lo-fi’s to hi-fi’s that I prototyped to assure the interaction design was sound.
Contextual report filters
Adding filters to show users about the data and overall system can offer.
AI feature grouping
Grouping call attribution data by how it is sourced to fit in user’s mental model of attribution as defined today
Acknowledgement of organic marketing
Calling out marketing growth that was generated for free. A lot of our target verticals receive referrals , so this is very relevant to their industry.
Conclusion
This was a very innovative project to work through. Outputs of AI need to be trustworthy, implemented within an existing workflow, and prove its value through reducing time spent on repetitive tasks to be something users take advantage of. I took consideration of that in my design decisions to assure users knew how AI was working for them.
How did it do? Success metrics:
Not yet shipped to customers, but here is what we are looking for:
SRA Report
Time spent on the SRA report (quantiative)
Clicks on the report filters and hovering over tooltips for education (quantitative)
Improved customer feedback on SRA in interviews (qualitative)
Increase in SRA attribution with tooltip after 90 days (quantitative)
Timeline
Time spent on the timeline / interaction cards
Engagement rate for key terms spotted / highlights